The Importance of Federated Learning in Web3 AI
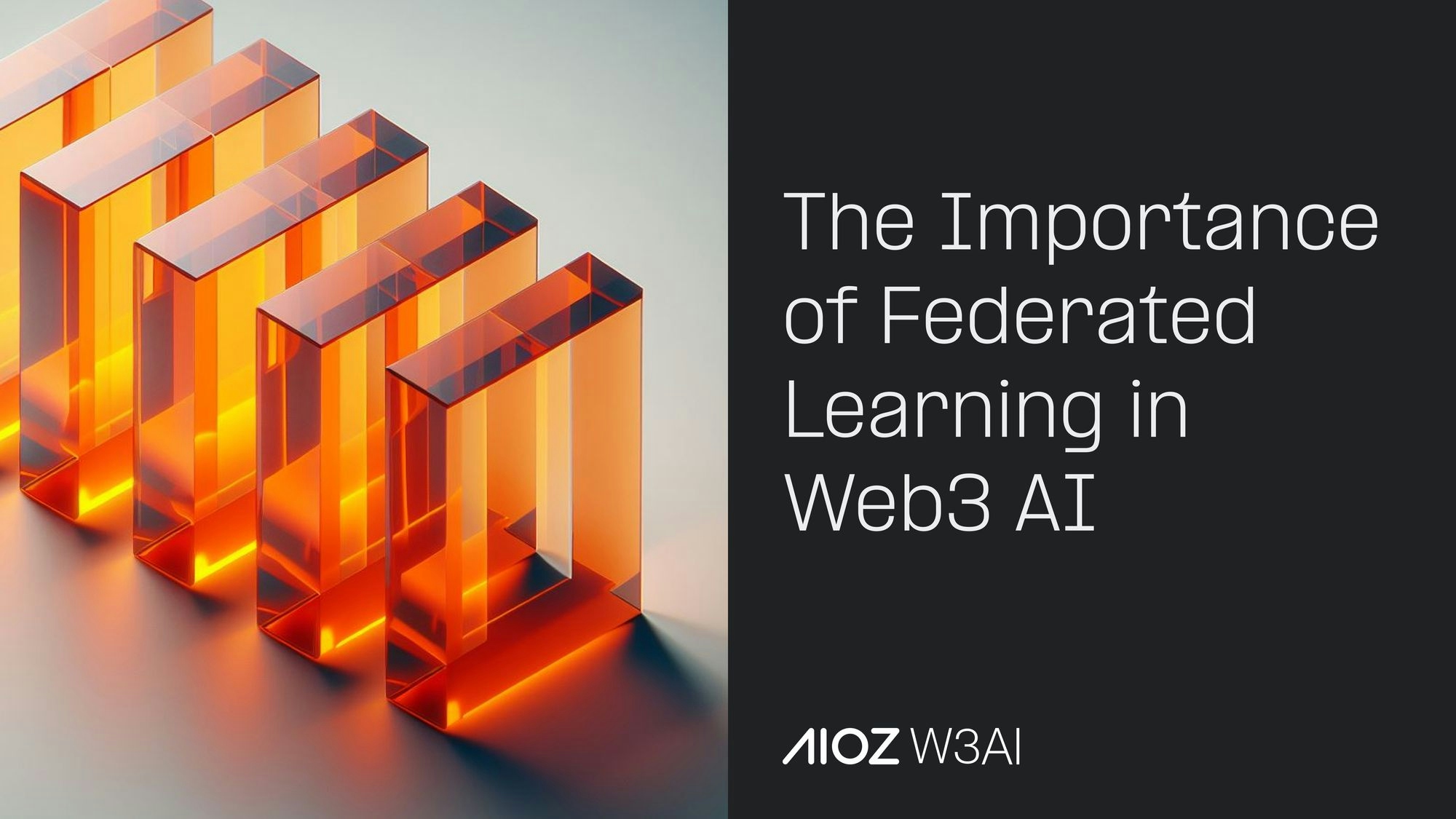
AI models are typically trained using single datasets stored on devices that belong to central authorities. These datasets comprise data gathered from various sources, including private/sensitive data owned by end-users.
While this AI training method is pretty straightforward, it has become increasingly difficult to execute due to the strict data privacy laws enacted in many countries.
This situation has led to the increasing adoption of an AI training method known as Federated Learning which enables AI models to be trained without compromising the privacy of data owners.
In this article, we will cover the Federated Learning training method and analyze why it is important in AIOZ Web3 AI (W3AI).
What is Federated Learning?
Federated Learning is an innovative training method that enables AI models to be trained on devices that belong to the owners of the training data.
As opposed to the traditional method where data is gathered from various sources and stored in a single dataset, with Federated Learning, local data stored on different types of devices (smartphones, PCs, IoT devices, etc.) can be used to train an AI model.
The rise of Federated Learning over the last few years has largely been feasible due to the increased processing power of consumer-focused devices that now have the ability to carry out AI computational tasks.
With Federated Learning, AI researchers can train AI models without the risk of breaking strict data privacy laws since the custody of training data remains in the hands of the data owners.
How Federated Learning Works
The Federated Learning process typically starts by distributing an AI model across all the edge devices containing local data that will be used for training the model.
After the training is completed, each device will upload its trained version of the AI model to a central model that aggregates all the different versions into a final version of the model.
The final version is then re-distributed across the same edge devices where it is fine-tuned with new parameters and re-uploaded to the central model for further aggregation.
This iteration is repeated multiple times until the final version of the model is fully trained and ready to move to the next stage in its lifecycle.
Federated Learning & AIOZ Web3 AI (W3AI)
AIOZ Web3 AI (W3AI) is a transformational AI-as-a-service infrastructure that is set to shake up the AI industry by ushering in a new era of decentralized AI computing.
W3AI will be powered by 80,000+ edge nodes in the AIOZ DePIN and will run on the recently released AIOZ Node v3 that enables these nodes to carry out Federated Learning.
Without Federated Learning, the concept of decentralized AI computing will probably still be a distant reality that members of the AI community can only look forward to.
However, thanks to Federated Learning, edge nodes in the AIOZ DePIN will be able to carry out advanced AI training tasks using local data, making decentralized AI computing a reality that is only a couple of weeks away.
This makes Federated Learning the backbone of the W3AI service which promises to liberate AI developers, businesses, and end-users from the stronghold that large centralized entities currently have on the AI industry.
If you would like to learn more about AIOZ W3AI ahead of its release, you can visit the link below to get a more detailed coverage:
https://aioz.network/blog/aioz-w3ai-shaping-the-future-of-decentralized-ai-computing

About the AIOZ Network
AIOZ Network is a DePIN for Web3 AI, Storage, and Streaming.
AIOZ empowers a fast, secure, and decentralized future.
Powered by a global community of AIOZ DePIN, AIOZ rewards you for sharing your computational resources for storing, transcoding, and streaming digital media content and powering decentralized AI computation.